
Mapping Marine Debris
Tracking The Rising Tide Of Ocean Plastic
Automating the identification of litter washing up on Hawaii’s beaches
That there is trash on beaches – even remote, otherwise pristine beaches – is an undeniable, and deeply unfortunate, environmental fact.
But figuring out a way to account for all of the debris that washes up on the beach, and ultimately a means to combat it, is a far less definitive effort. Consider Hawaii, which is exposed to currents that flow through the Great Pacific Garbage Patch, and has not only a lot of beaches across the islands – 137, all told, some of them very small – but many very remote stretches of coastline. Could the debris on every single mile of coastline be both documented and cataloged? In 2015, two researchers in Hawaii undertook a project to attempt just that – setting off on an on-going research effort that could result in a powerful tool for coping with marine debris.
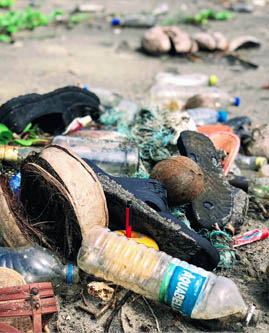
Detritus, Bhogave Beach
John Cameron
“Collecting the imagery is one feat, but that actually is the easy part,” said Miguel Castrence, a remote sensing scientist who collaborated on the project with Kirsten Moy, a graduate student at the University of Hawaii, Manoa’s department of geography and the environment. “The hard part really is analyzing it.” Doing so the old-fashioned way, by looking at each image and marking and identifying pieces of debris of all shapes and sizes, was exceptionally hard: it took eight analysts 688 hours over the course of 3.5 months to analyze images taken of all 1,223 miles of Hawaiian coastline, identifying over 20,000 items. Debris ran the gamut from objects the size of a shipwreck to items as small as a bottle of detergent. But while analyzing the data was a significant accomplishment, countless new pieces of debris of course washed up on the shore during those eight months, making the analysis an impressive exercise that could not effectively be utilized in the field or easily replicated.
One of the analysts, who were all student interns from UH, asked Castrence why the process wasn’t automated – a question that stuck with him, even if he knew the various reasons why. “The simple but unsatisfactory answer is that we didn't have the time, funding, and knowhow,” Castrence said. But a few years later, he and colleague Kirsten Moy decided to redo the analysis using machine learning, putting the work of those interns on the back of a computer algorithm. Their project, Mapping Marine Debris, was one of the 20 finalists in Round 1 of Conservation X Labs’ prototyping competition, the Con X Tech Prize.
Project Snapshot
Mapping Marine Debris
Other
Garbage In, Marine Debris Info Out: Doing the dirty work of image analysis using machine learning and citizen science
Not accepting new members
With both the coastline image data and the interns’ analysis of the debris, the pair had a solid basis for an algorithm – they just needed someone to write it for them. And they found the ideal person through the Digital Makerspace. Yutaka Hosoai, who has a day job as the lead data scientist for Coupa Software, was looking to volunteer his skills for a marine conservation project, and found Castrence and Moy on the Digital Makerspace.
Amazed by how perfect of a fit Hosoai was for the project, Castrence says that other projects working on the Digital Makerspace should be open to finding new collaborators from the outset. “We would strongly recommend that other project instigators have a plan for potential collaborations and volunteers so they can immediately put eager minds to work,” he said.
While the plan had been to take the human analysis, have Hosoai build an algorithm using it, and then re-run the analysis with the computer doing the work, the team ran into some issues with how a human identifies and recognizes a piece of trash and how a computer sees the same images. “We thought we could just dump the data in and the machine learning would automatically be able to handle it,” Castrence said. “But we found out that there were significant discrepancies between how the data were identified.” While the students often marked debris with a dot – sometimes in the middle of a piece of debris, sometimes off to one side – the algorithm needed a square to demarcate what was trash and what was not.
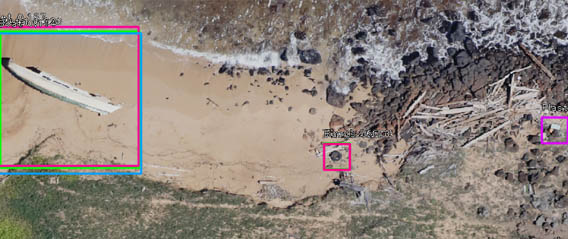
Aerial imagery with vessel and other debris identified by algorithm
Resource Mapping Hawaii
The machine-learning analysis is still ongoing, with roughly half of Hawaii’s coastline currently mapped. (The current hangup is related to the platform the team is using for image annotations, and once it’s resolved a number of volunteers should be able to finish the human labor required to let the algorithm complete its job.) And while the team still wants to see how the computer analysis compares with the human one, they’re also thinking about how this tool could be used for conservation purposes.
“We can detect something, but we can't fly an airplane every day,” Hosoai said. “How can we get new images efficiently and detect it? Once we detect it, what can we do with it? Are we going to notify someone?”
These questions remain to be concretely answered – and with some micro grant money left from the competition, Castrence said they may hire some more interns as the next steps for the project are determined. But Moy imagined some compelling applications that, considering where their research is at the moment, could be quite close at hand.
“Think of a beach cleanup where you have a small group of volunteers, all of them dedicated and they are collecting the debris off the beach,” she said. While part of the work is removing the trash, there’s the necessary – and more laborious – process of sorting, bagging, and weighing it. Because if you don’t know what trash was there, and what’s been removed, the beach may be cleaner but there’s been no contribution to the broader understanding of how what debris ends up where and in what quantity – or give any direction as to where to put pressure on the industries that are responsible for the various kinds of debris in the first place. With their algorithm, a beach clean up might look very different. “If we could simply have someone fly a drone down the coastline first,” photographing the coastline and using machine learning to scan the debris, “then you don't have to do any of the manual sorting and documenting,” Moy said – a significant step in the right direction.